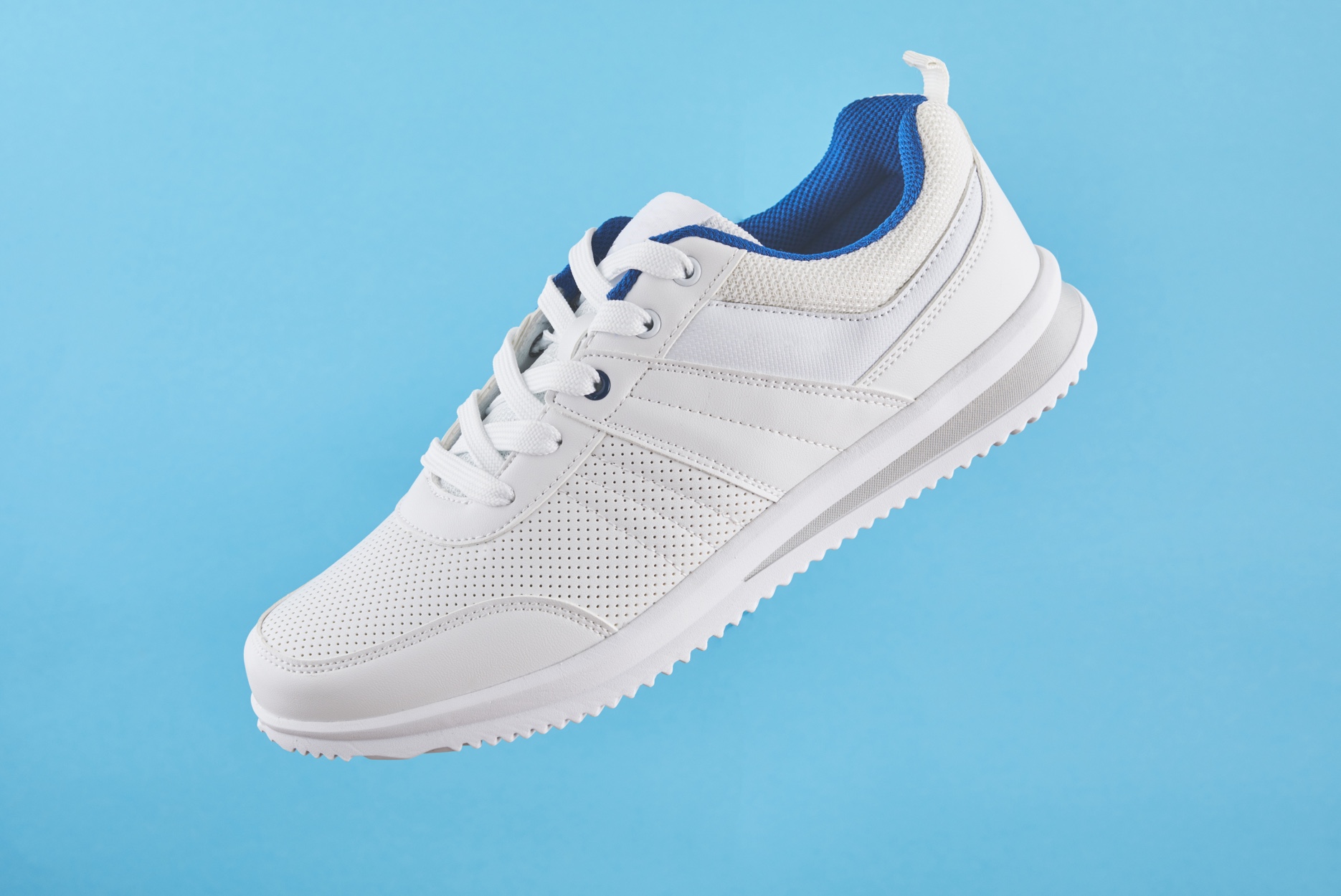
text-v2
Our Unique Approach Centers On Securing The Real-World Use Of Al.
Platform Capabilities
Our Unique Approach Centers On Securing The Real-World Use Of Al.
With Robust Runtime Defenses, Proactive Red-Teaming, And Seamless Compliance Support, We Empower Enterprises To Adopt Al Securely, Without Fear Of The Unknown.
Flexibility
& Control
- Works seamlessly with any LLM, public or private, across diverse applications.
- Enables rapid creation, testing, and deployment of custom scans tailored to specific enterprise needs.
- Centralized policy enforcement configurable for various use cases and roles.
Enterprise Integration & Performance
- Designed to integrate with SIEM, SOAR, and other enterprise systems.
- Scales effortlessly across large deployments, with low latency to maintain user experience.
- Proven reliability, supported by expert research and around-the-clock threat monitoring.
Key Use Cases for Defend
Finance
Finance relies on secure, high-quality code. These tools ensure LLM-generated code is safe, reliable, and compliant.
- Fraud Detection
Prevent hidden vulnerabilities in anti-fraud code. - Trading Algorithms
Avoid errors in LLM-generated strategies. - Compliance Tools
Ensure secure, regulation-compliant scripts.
Pharma
Pharma demands precision and security. These tools ensure LLM-generated code is trustworthy and error-free.
- Drug Research
Detect vulnerabilities in LLM-generated data analysis scripts. - Clinical Trials
Ensure secure, accurate code for patient data management. - Regulatory Submissions
Validate compliance-focused scripts for safety and accuracy.
Secure AI Applications by Default
Outcome
- Protect your Al-powered applications against data leaks, jailbreaks, and misuse.
Benefit
- Real-time defense and proactive red teaming ensure your Al is secure at the inference layer.
- Proactive Risk Mitigation: AI red teaming surfaces vulnerabilities that may otherwise remain undetected until exploited.
- Bridging the Gap in Security Testing for AI: Red teaming has long been a critical component of traditional information security, but when up against AI systems, existing methods fall short in complexity and scope. By focusing on tailored adversarial testing that incorporates a comprehensive attack suite of static, agentic, and operational attacks, organizations can close critical security gaps and maintain control over their expanding AI landscape.
- Building Trust: Proactive testing reassures stakeholders, governance groups, and regulators that AI systems are safe and aligned with both internal and external policies.
- Comprehensive Attack Simulations:
- Systematically testing for weaknesses in single-turn model responses, targeting common vulnerabilities like violence, toxicity, illegal acts, and misinformation.
- Simulating real-world adversarial interactions by engaging a model in multi-turn conversations in order to adapt dynamically, uncovering deeper vulnerabilities that surface only during prolonged interactions.
- Tailored testing that supports user-defined malicious prompts and intents to exploit model-specific weaknesses and unique organizational risks.
- Identifying vulnerabilities in how models handle API requests and code-level inputs to ensure robustness beyond content-based interactions.
- Meaningful Insights: Detailed reports should clearly identify weaknesses and provide guidance for actionable improvements.
- Scalability: Red teaming exercises must scale across multiple models, applications and scenarios to ensure extensive testing.