- Ensuring the accuracy of the information provided, including whether it is free from obvious and inherent biases (or hallucinations)
- Ensuring the validity of the information, including whether it is free from malicious content and meets the organization's quality standards
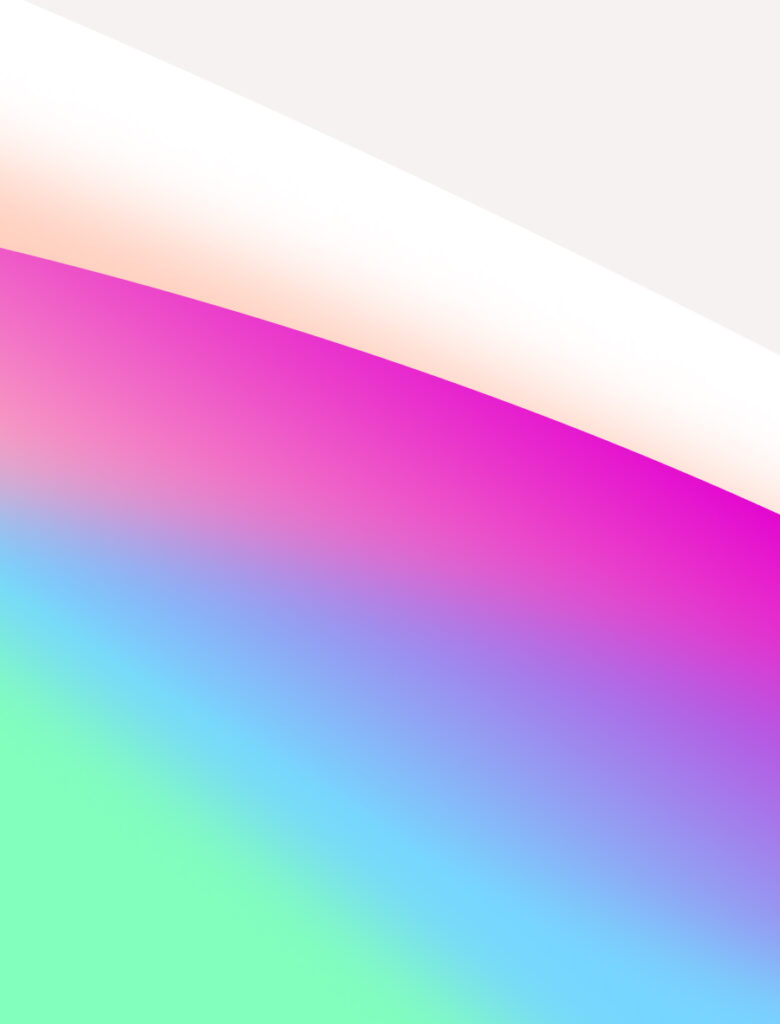
Blog
08 Mar 2024
LLMs: The Good, The Bad, and The Ugly
LLMs: The Good, The Bad, and The Ugly
LLMs: The Good, The Bad, and The Ugly
It’s been a little over a year since generative artificial intelligence (GenAI) and large language models (LLMs) captured the world’s attention. Black hats immediately fell in love with them because the number of possible threat vectors increased exponentially. White hats fell in love with them because they held (and continue to hold) such tremendous promise for bringing advances to everyday life, such as speeding up the transactions and interactions that can make life better, like credit decisions and job offers, as well as for truly life-changing achievements, such as developing and testing new medicines, therapies, and procedures.
And although many organizations have taken advantage of the benefits of GenAI by deploying models across the enterprise to tremendous effect, such as increased productivity and streamlined operations, many more have not. The reason is they hold a genuine and not unfounded fear of things going terribly wrong, from cost and deployment miscalculations to employee misuse to data or system breaches. Gaps in the AI security apparatus, after all, can result in tremendous fallout from intellectual property or other sensitive data being shared via poorly written prompts or weak system safeguards, or malicious code making its way into the organization via unmonitored responses. However, there is an equal and opposite fear facing these hesitant decision-makers: getting left in the dust of mere automation as competitors ramp up their deployment of GenAI and AI-dependent systems, such as chatbots.
Both groups—the enthusiastic early adopters and the foot-draggers—face the same two critical business risks that have yet to be fully addressed by the model providers: